Machine
Learning & AI
We help you grow your business by implementing intelligent technology.
We grow your business
with intelligent technology
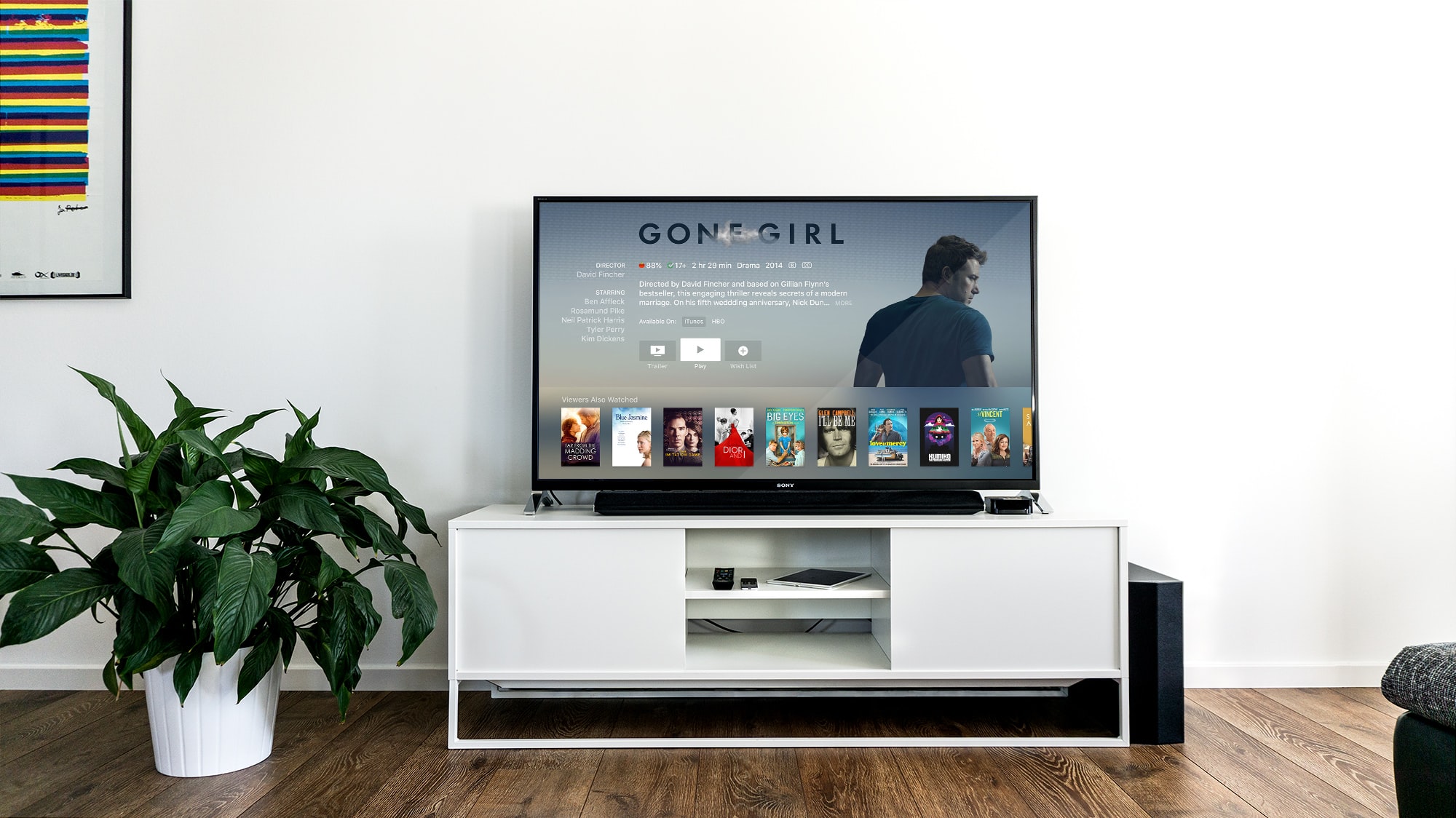
Recommender Systems
Recommender systems are used to offer users products or services based on their preferences and behaviour.
Example
Netflix knows what you'll like based on the other shows you watched.
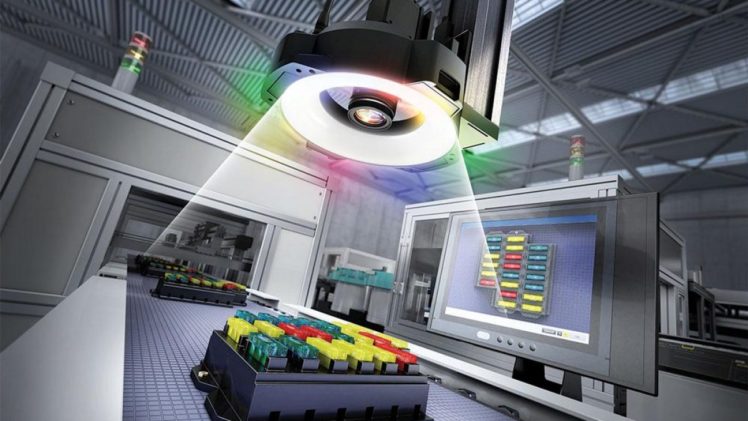
Visual Quality Control
Quality Estimation uses machine learning models to assess the quality of a particular product or resource via Computer Vision.
Example
Domino’s Pizza Checker is a video control system that verifies that pizzas look the way they are supposed to. It measures size, shape, distribution of ingredients.
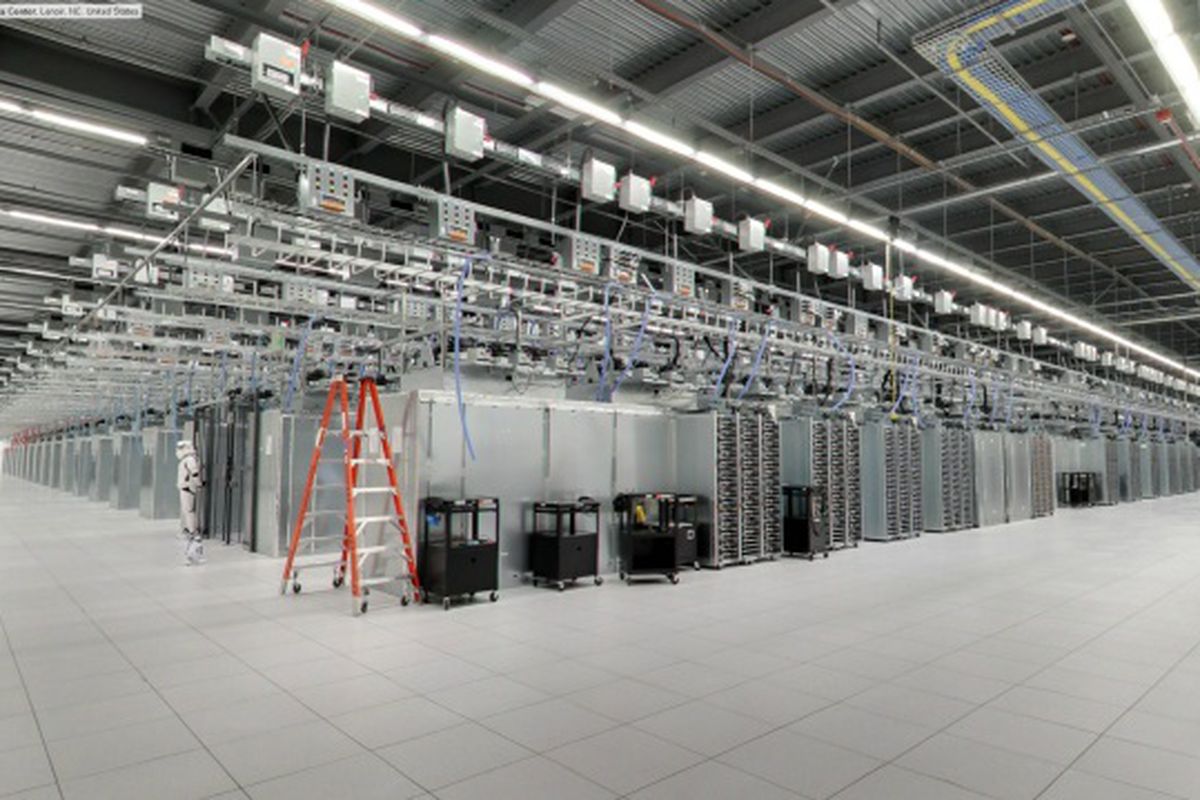
Continuous Control
Deploy a Reinforcement Learning agent to control a machine or environment continuously.
Example
Algorithms in Google's data centers autonomously control cooling systems by matching computing power with the weather, thereby saving costs by 40%.
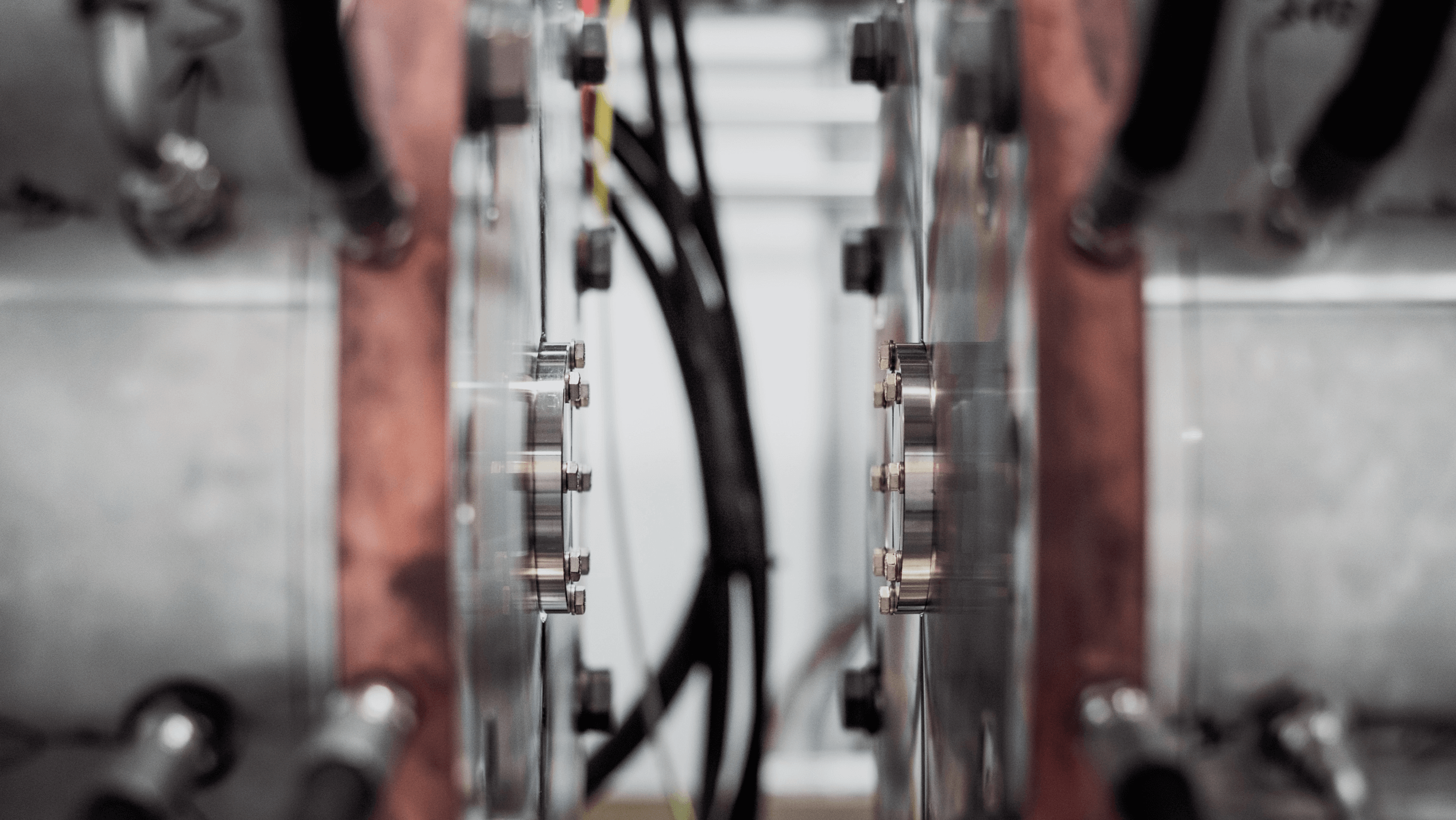
Automatic Calibration
Use a Reinforcement Learning agent to support the calibration of a complex machine via a feedback mechanism.
Example
Microsoft and Siemens reduced calibration of industrial machines from 40 hours to 1 hour.

User Clustering
User clustering divides individuals into groups based on shared characteristics that are often too complex to detect by a human.
Example
Marketeers cluster their users into personas and create unique marketing campaigns tailored towards each user group.
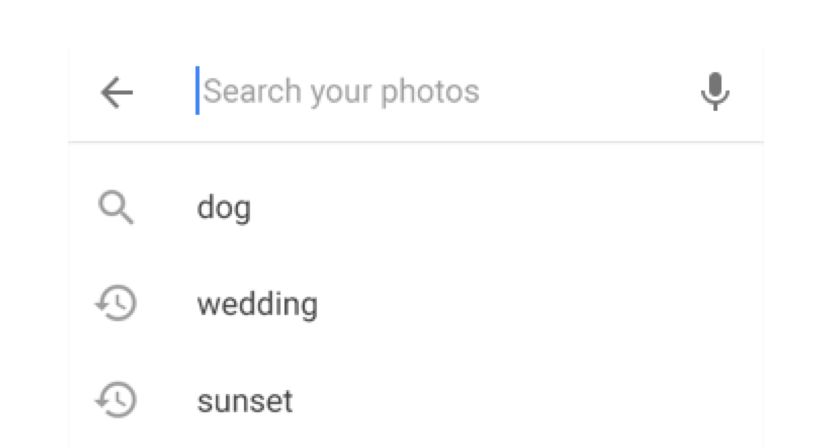
Image Classification
An Image Classifier is a computer vision model, capable of assigning an image to a specific content category.
Example
The AR application we created for Woody can recognize specific animals on the pyjamas.

Churn Prediction
A Churn prediction model is used to predict which customers are likely to cancel their subscription or consumption of a product.
Example
Netflix uses Churn Prediction to detect when a customer is likely to cancel the subscription.
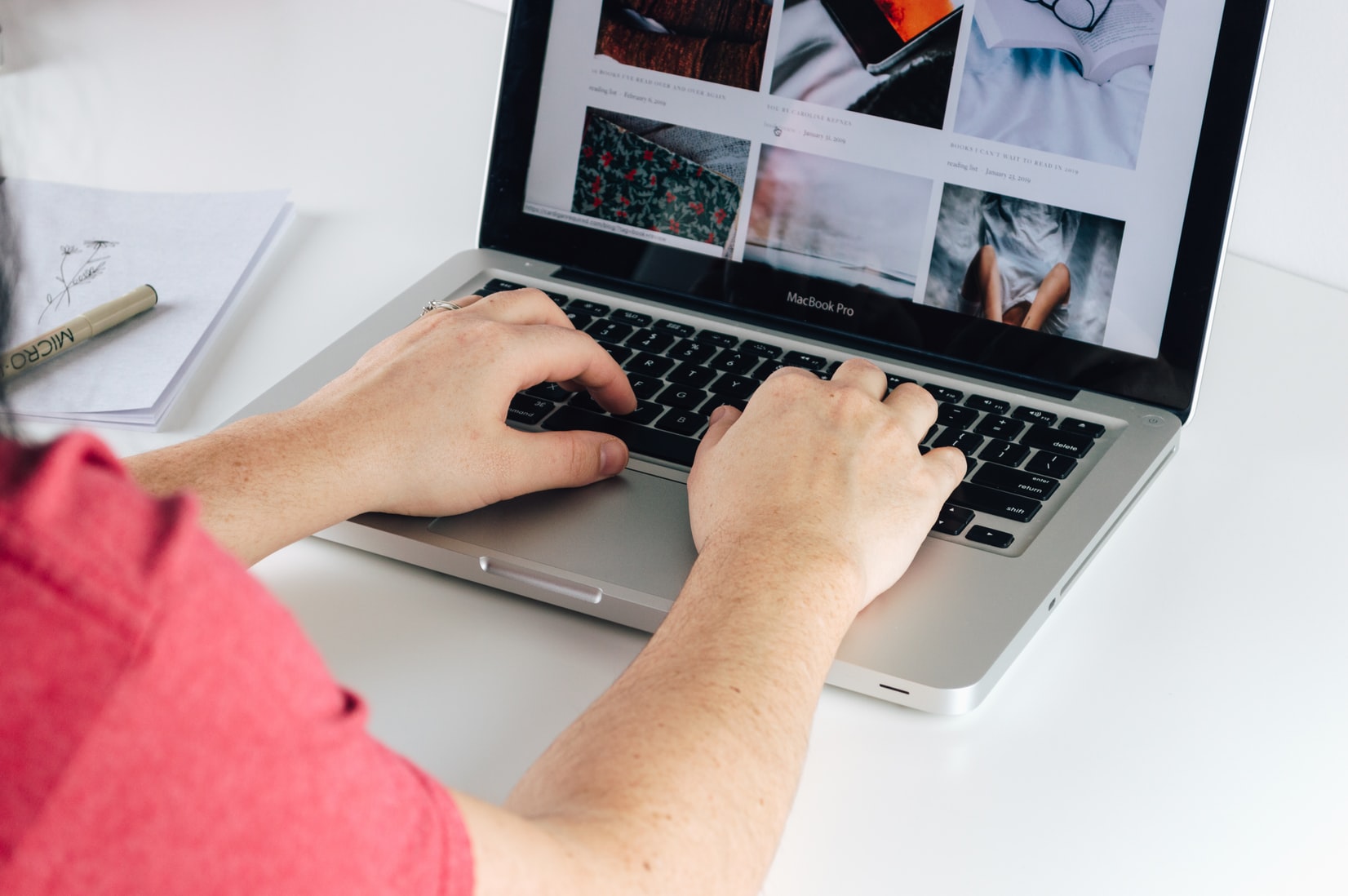
Text Classification
Text Classification is the task of assigning predefined categories to the new text.
Example
We created an automatic mail classifier for SD Worx that is able to read emails and understand what the content is.
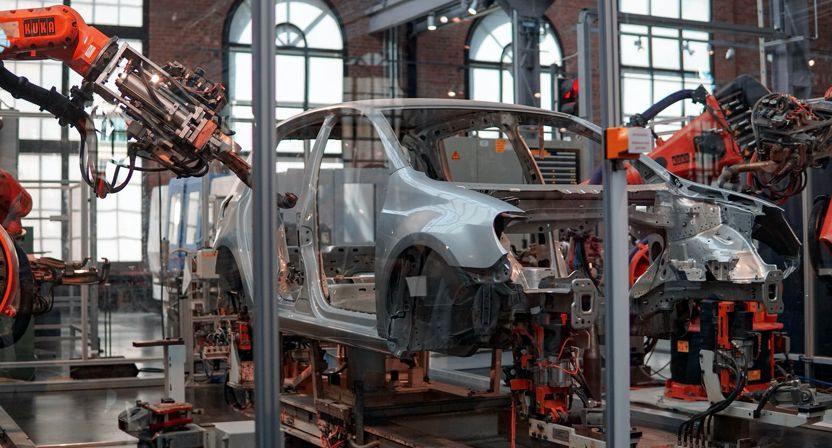
Predictive Maintenance
Predictive maintenance can anticipate when a machine is likely to fail soon. From this, you can prioritize your planning and prevent downtime.
Example
Bosch equips machines with sensors to monitor vibration and uses this information to predict the deterioration of a machine and suggest maintenance.
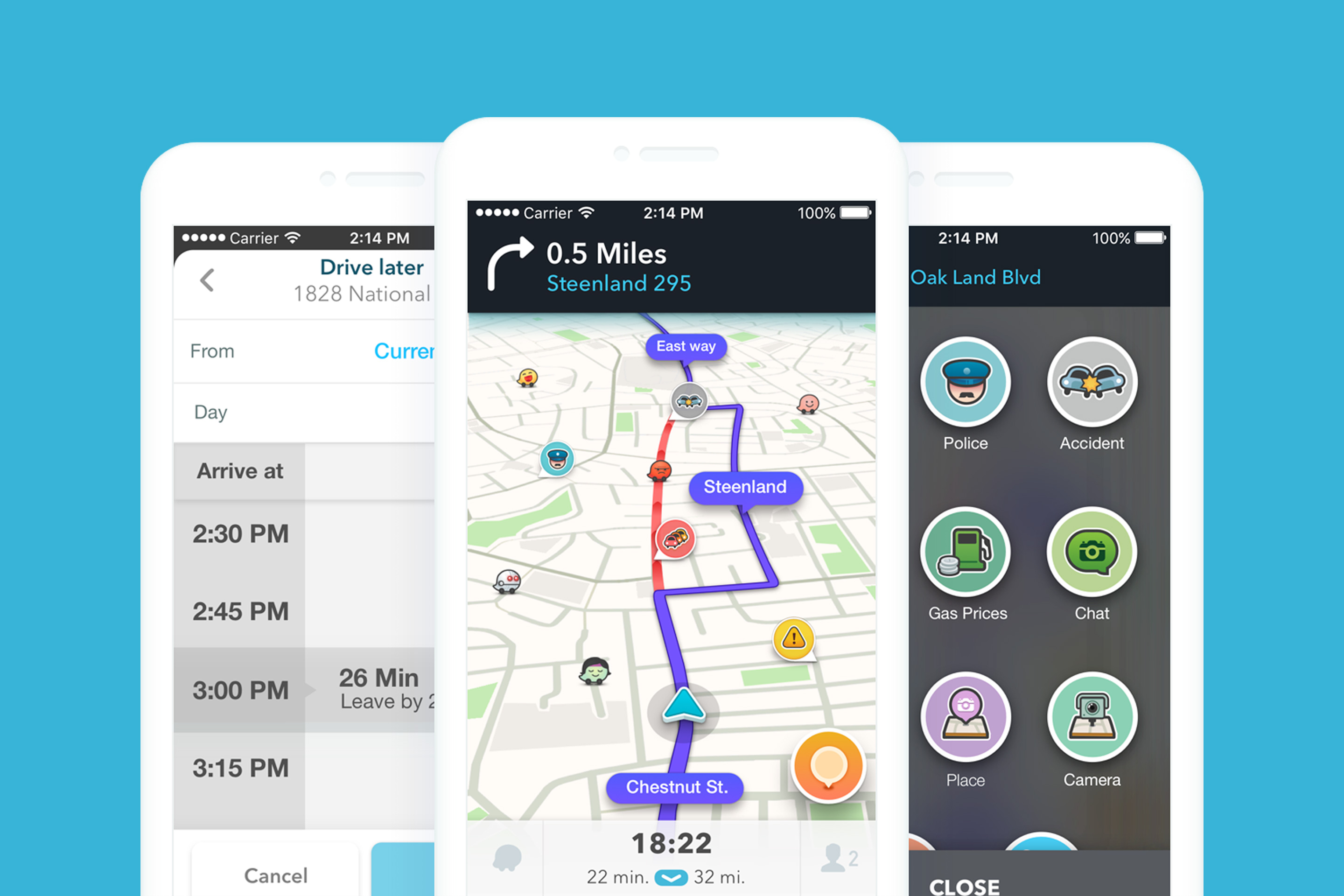
Time Series Trending
Time Series Trending utilizes methods for analyzing time series data to extract meaningful statistics and other characteristics of data.
Example
Waze and Google Maps use time series trending to anticipate traffic jams.
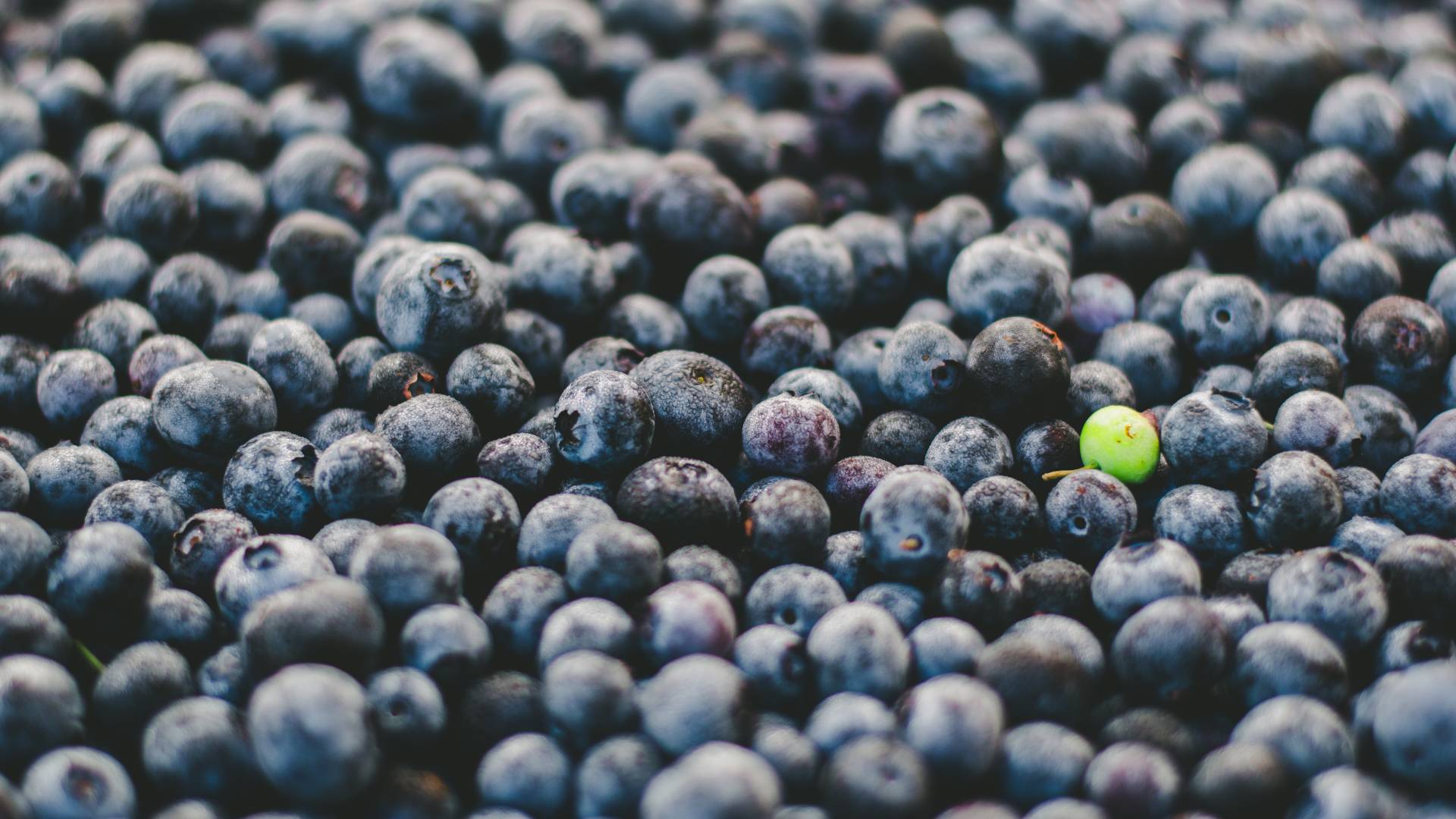
Anomaly Detection
Anomaly Detection is the identification of rare items, events or observations that differ significantly from the majority of the data.
Example
Lemonade is an insurance company that uses an Anomaly Detection algorithm to spot fraudulent insurance claims.
Why In The Pocket?
We're a Digital Product Studio
We do not solely consist of AI engineers. We’re a digital product studio with more than 110 strategists, designers, engineers and data scientists. We have 10 years of experience in building fully integrated digital products that millions of people use.
We deploy to any platform
Our cross-functional teams exists out of a mix of competences. Mobile, Edge, Web, Cloud and APIs, we deploy to any platform that is best suited to address your needs and we are always up to date with the latest developments.
Focus on human centered AI
AI operates in the sweet spot between business, technology and humans. You need to solve a customer need and a strong focus on best-in-class user experience. That’s where our experts come in.
Proud to be official Google Cloud Partner. With Professional Google Cloud Certified architects and data engineers in all of our platform teams, we make sure that we leverage the full potential that Google Cloud has to offer and are sure that the choices that we make are based on solid insights.
How to get started
AI Discovery
A first introduction to what AI could mean for your business. Download our free canvas.
- Half day workshop
- AI Card Game
- AI Value Canvas
AI Prototype
A prototype for your most valuable AI use-case to immediately see its value in action.
- Identifying the opportunities
- Building the pilot
- Creating your roadmap
AI Implementation
A cross-functional team that builds and ships your product in no time.
- Dedicated cross-functional team
- Continuous development
- 2-weekly demo’s