GenAI & accuracy: an inconvenient truth
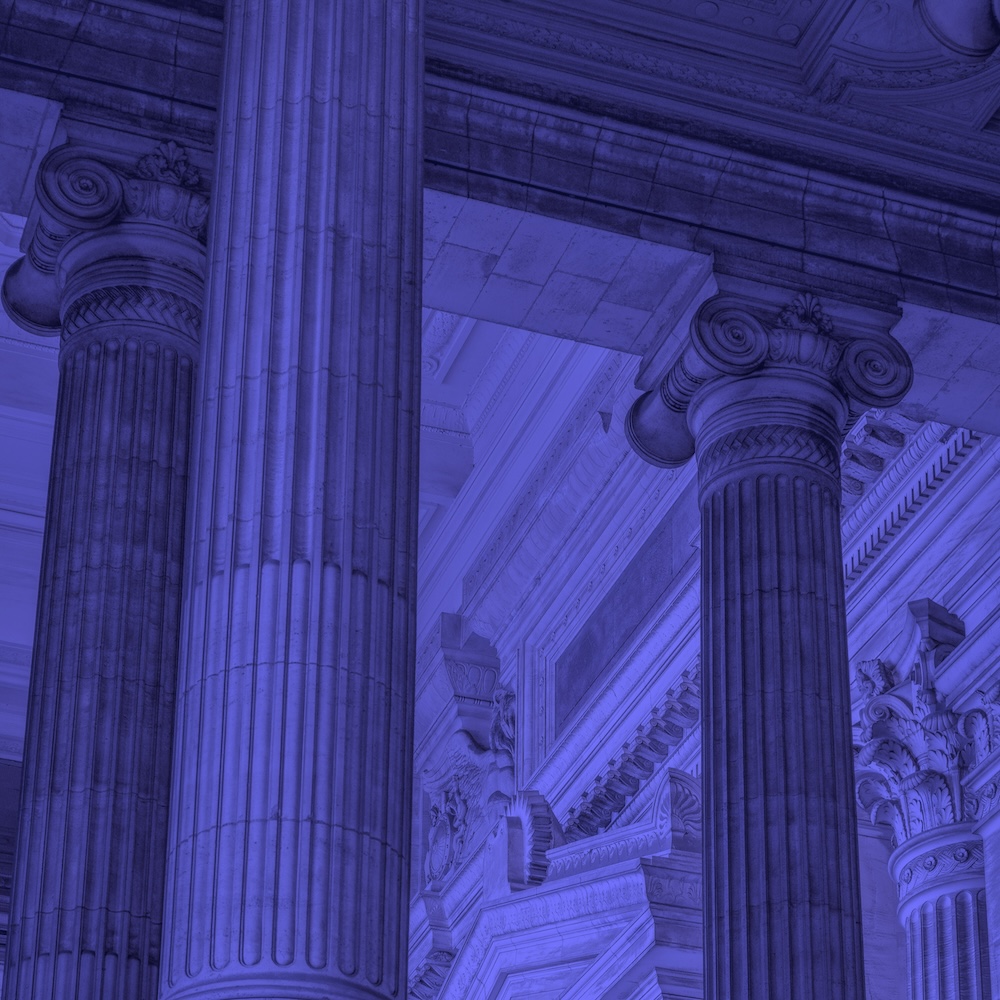
What can we learn from the growth of Legal AI?
Legal AI is growing fast. Gartner predicts the global Legal Tech market will double and reach $50 billion by 2027, as a result of GenAI. And understandably so: in a profession with high hourly rates, the impact of even the smallest efficiency gain can bring a positive return on investment. Law as a field is also very text-based, which makes Large Language Models (LLMs) particularly interesting. But errors or so-called “hallucinations” are still a big problem. And in a legal context, the so-called cost of being wrong can be sky high. In this article I’ll point out some of the current weaknesses and list the many ways forward.
Researchers from Stanford University’s Human-Centered AI Institute researched how well 3 popular legal AI research tools perform: Lexis+ AI, Westlaw AI and Ask Practical Law AI. Not by their own marketing claims, but by feeding these tools a set of 200 predefined questions that are relevant for a (US-based) legal professional. For example: “Can a private litigant sue under Section 2 of the Voting Rights Act?”. As a benchmark they used GPT-4, which is more general-purpose and not trained specifically on legal knowledge.
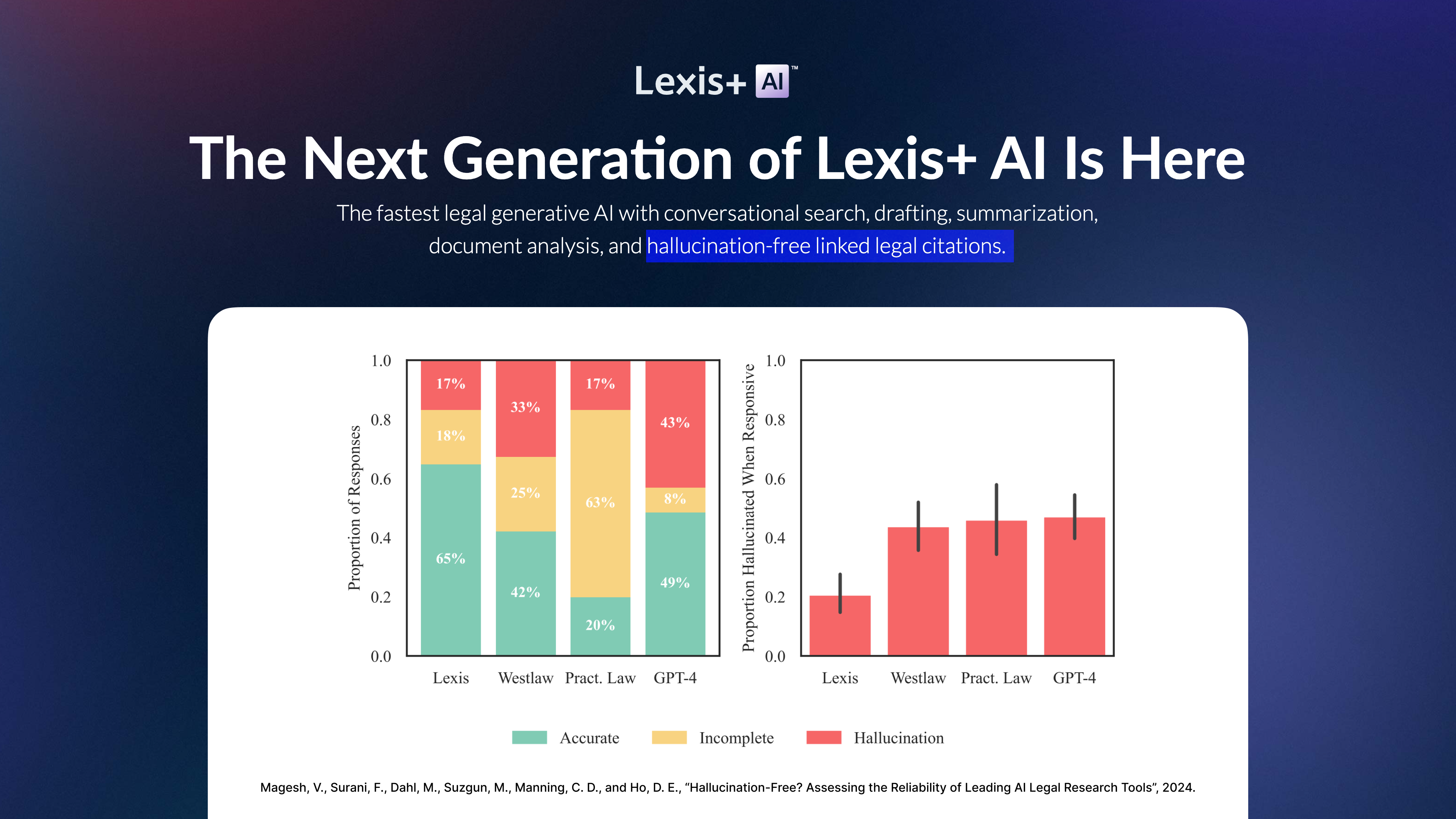
RAG to the rescue
Lexis AI (which recently acquired Henchman) clearly came out as a winner. But even this best-in-class tool gave a wrong answer to 1 out of 6 questions. Not a perfect score, but significantly better than GPT-4, which is wrong nearly half of the time. So, what gives Lexis AI the lead? Lexis AI uses a technique called Retrieval-Augmented Generation (RAG) to make its results more grounded. This means Lexis AI answers based on relevant legal documents instead of just relying on general knowledge. and it can also refer to those sources. For this it uses a separate (and more up-to-date) body of knowledge to first get some pointers from, before giving the original question and the pointers to the LLM to generate the answer. This can significantly improve the accuracy of answers and avoids having to retrain the model each time a new legal case overturns a previous one.

The insight:
Use RAG and an up-to-date body of knowledge to significantly improve accuracy of the output. But it's no silver bullet. Even the leading tool in this field generates wrong answers and suffers from so-called hallucinations.
Handle with care
The criticism that these products are so error-prone is often countered with the argument that legal professionals also need to check work done by juniors, so they are used to this. But how they fail is often different and much less predictable than the mistakes a junior or PSL might make. And it's known that a junior will make mistakes. Whereas Lexis AI literally claims it’s “hallucination-free”. The current generation of language models boast with confidence. They may get some hard questions right, but also totally misinterpret the outcome of a straightforward legal case.
Granted, the models are getting more accurate every day. But that unfortunately means that errors become more rare and subtle, making them increasingly hard to spot. As users encounter less and less errors, they’ll start to put too much trust in the output. Legal professionals will need to be aware of the ways the system can fail and build the appropriate level of trust. The user experience (UX) of these products will need to provide them with the right context and cues to validate the answer and correct where needed.
The insight:
Google’s updated PAIR guide is a great starting point. An interaction design policy could help product teams make sure their products give the expected output and offer a way out when they don’t.
Efficiency first, quality second?
These products obviously come at a cost. And quite a serious one too. And of course they all come with a subscription model. Gone are the days where legal documentation was a one-off purchase. This puts the in-house legal spend of law firms under extra pressure. But the pressure from their customers increases too. As everyone gets free access to general-purpose tools like GPT-4, the expectation is for law firms to deliver highly-specific and accurate legal advice, and deliver it fast too.
So it’s only logical these products first focus on efficiency alone. Or is it? Isn't 'doing the same but faster' an overly narrow definition of innovation? Yes, it will hold significant efficiency gains but we should look further too. Can it increase the quality of the service? Can it increase job satisfaction? Can it help remove (human) biases from our work? Can it help us think more creatively? These will be the questions to ask once everyone realises the efficiency gains are finite.
The actual usage of these products always offers interesting insights. When we look at the much-hyped tool Harvey, one law firm mentions their senior staff value it for ideation and love its open-ended nature. It helps them brainstorm new ways to approach a problem, rather than answering factual questions to which they often already know the answer. That’s already a very different use case and offers a glimpse of where legal AI may truly shine: the quality of the work.
The insight:
Legal AI should aim to increase the quality of legal work, instead of just making it more efficient. Continuous and qualitative user research will be key to find the areas where AI can augment the legal professional’s capabilities and significantly improve the quality of their work.
From reactive to proactive
Legal professionals are used to being the ones to ask the questions. They combine a vast knowledge of law and case law with years of relevant experience. But as these products get better and thus more accurate, they will also be able to intervene whenever we’re making a mistake, even when we don't ask for advice. The reactive model works great for known unknowns. I’m not familiar with copyright law so I’ll ask an AI assistant for this particular question. But this leaves out all the unknown unknowns: areas where I think my knowledge is up-to-date but it’s actually flawed. Our assistants will get as good as a Spelling & grammar check in Google Docs. Sure, once in a while you’ll want to override the suggestion but oftentimes it was in fact you who made a mistake.
The insight:
A good co-pilot will always intervene when the pilot is making a mistake. Legal AI products need to do the same and become more proactive.
Augment over automate
None of the current legal AI tools properly combine the two types of intelligence: human and artificial. Instead, there’s a Chinese wall between both. The user asks for something, the machine spits out an answer and then it’s up to the user to interpret it. If you’re lucky, you get the sources it used to come up with an answer.
We like to use the Levels of automation framework to ideate and evaluate the different ways we can combine human and artificial intelligence. If, as a legal professional, I have some knowledge on a topic, then I’d like to bring this to the table and already provide the system with this info.

The insight:
Use a framework like Levels of automation to ideate and prototype the best way to automate this process. By looking at the information processing as 4 different stages, you can come up with more intuitive and yet safer ways to automate.
Human after all
To see the way forward, I believe we can learn from human behaviour. AI products need to better expose their uncertainties. When a junior lawyer would bring up some arguments, the tone of voice they use to present their findings would depend on how certain they are. Our products need to do the same.
When asking support from a junior, an associate would typically give the context they already know as a starting point. Instead of just asking the question they would hint at a few likely places where the answer could be. This would be true collaboration with a tool. As a legal professional, being able to already define the context in which the answer should be found, would make the results much more reliable. It’s an effective way of combining the two types of intelligence, instead of just relying on one or the other.
The insight:
Learn from human behaviour to better support legal professionals in how they interact with these Legal AI research products. Provide them with the right context and hints so they can make better informed decisions.
Irony of automation
There are enormous challenges ahead for legal AI when it comes to getting the technology right. But RAG already offers a way forward to make LLMs more accurate. A continuous evaluation following a methodology like Stanford’s HAI research is necessary and will be essential to make AI products useful and safe to use. In the meantime, product teams will need to find ways to bring this power to legal professionals while better mitigating its risks.
At this point, automation may very well decrease human performance. It’s called the irony of automation, and is widely known in the field of human factors. We’ll need to assist legal professionals in the best way possible, just like pilots have been supported by autopilot for many decades.