How can we align the AI of the future with human values?
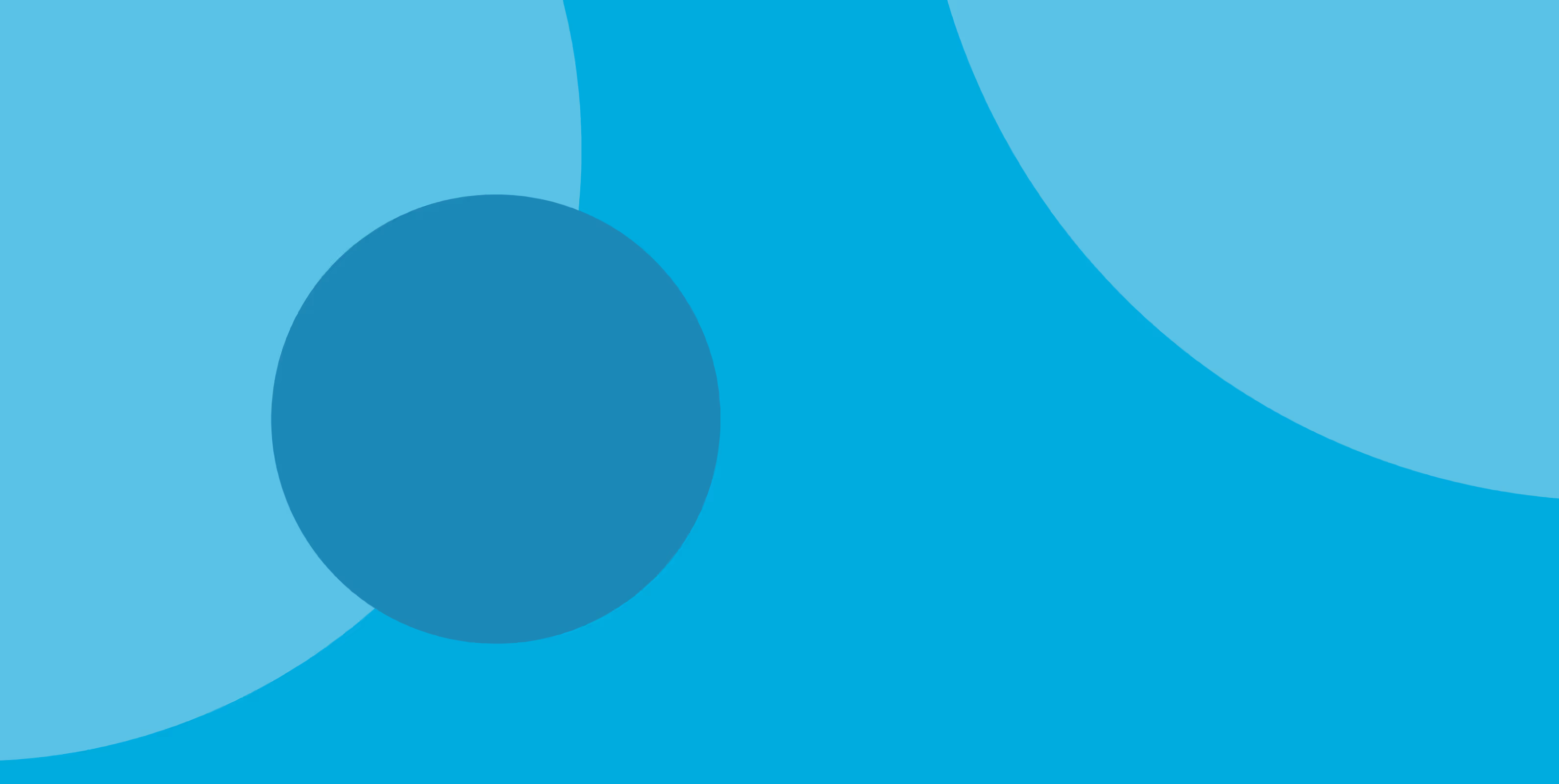
We recently participated in an intriguing AI safety workshop organized by the Effective Altruism movement, where the long term consequences of creating increasingly intelligent agents were discussed. How large are the risks involved in continuing the rapid development of artificial intelligence (AI)? Even today, it is already clear that introducing powerful AI algorithms to the world requires considerable vigilance.
The field of artificial intelligence has experienced an impressive renaissance this decade. With tour de forces like DeepMind’s AlphaGo defeating 18-time Go world champion Lee Sedol, or AlphaStar defeating one of the world’s strongest StarCraft II players, it seems like there is no stopping the advance of intelligent algorithms becoming superior to mankind at increasingly complex tasks. One could wonder if this general trend will continue to the point, ominously called the singularity, where machines effectively surpass man’s abilities in every imaginable aspect. Indeed, why would the intelligence of homo sapiens be the last frontier? It seems highly unlikely that this stage in human evolution represents the summum of intelligence, unsurpassable by any other form of intelligence, artificial or otherwise. Although we are probably still not close to building a full-blown artificial general intelligence (AGI), the very idea that this is likely to happen in the not so very distant future (experts predict a 50% probability of high-level human intelligence by 2040–2060) should give us some pause and have us reflect on how that scenario would play out for us. How do we make sure that a future superintelligent algorithm helps us achieve our goals and dreams, instead of unintentionally eliminating us in the process of fulfilling its own purpose? This problem is called the value alignment problem, and it most definitely needs addressing before we are to introduce the second form of general intelligence to this world.
It seems highly unlikely that this stage in human evolution represents the summum of intelligence, unsurpassable by any other form of intelligence, artificial or otherwise.
Flawed rewards and crashing cruise ships
The danger of switching on a powerful superintelligent agent is not that it will all of a sudden become sentient and plot a scheme to eliminate us all out of spite and revenge for all the bad things we’ve done to machines in the past. Machines needn’t have consciousness or feel emotions to pose a serious threat to us, and their purpose needn’t be the extinction of humankind. The real inherent risk comes from the consequences of the flawed machine learning paradigm that we use to have an agent obtain the goals specified by us. The flawed, but up until now very successful paradigm is the following: if we want an agent to learn how to achieve a certain task, it gets rewarded when it completes that task or shows behavior towards completing that task, and it is punished (negative reward) when it fails to meet its objective. The reward for being in a particular situation is specified by the so called reward function, and the agent’s objective is to maximize this function. Throughout its training, an agent learns which situations (caused by which actions) bring about a high reward, and which don’t. After a while, the agent gets really good at maximizing its reward function and it now has hopefully learned to do what we wanted it to do.
Let’s go through an example of the paradigm; say we want to have a humanoid robot learn to stand on its two feet. We might implement the reward function as having a higher value when the head of the robot is further removed from the ground. At first, the robot is clueless as to what it’s supposed to do, but after some trial and error it notices it gets larger rewards from stretching its legs or torso upwards. After many iterations of training, it now is able to fully stand up, almost consistently maximizing its reward function. Notice that the reward function provided here is a proxy for the actual goal; it is cleverly engineered as to provide us with behavior that can be considered as ‘standing up’. Sometimes, however, an implemented reward function does not lead to the desired behavior, and the agent acts in unintended ways. A simple example of this is a case from OpenAI, where a reinforcement learning agent had to maximize its score in a boat racing game. Instead of becoming a superhuman boat racer that is able to finish the race in incredible times, it maximized its reward function by going in circles, infinitely hitting intermediary bonus targets (while even crashing into walls), not even trying to finish the race.

Larger in scope are the algorithms that social media use to maximize their content click-through (or maximize view time, or similar). The underlying idea (naively) might be to offer as much value to customers as possible, but by using an easy proxy for the real goal such as maximizing click-throughs or viewing time, things like echo-chambers and the pushing of extremist views are the actual results of this imperfect reward function. This reward function unintendedly influences things as important as presidential elections or national security.
Nespresso, what else?
It is clear that even the relatively ‘dumb’ algorithms social media use have a significant impact on our society. As these algorithms become more and more intelligent, they will most likely become even better at maximizing their reward function. If this reward function remains as flawed as before, the algorithms will easily manipulate our way of thinking, probably without us even noticing. Stating that we should simply implement better reward functions that don’t have unintended side effects is much easier said than done. Every direct hard coded reward function that we come up with will almost certainly have unintended side effects when implemented in a superintelligent agent. Even worse, once a superintelligent agent is turned on with such an engineered reward function, we cannot turn it off when we notice it shows unexpected and unwanted behavior.
Every direct hard coded reward function that we come up with will almost certainly have unintended side effects when implemented in a superintelligent agent.
To see why, let’s imagine you ask your superintelligent robotic house servant to make you a cup of coffee. If you, for some reason, want to halt the robot from making that cup of coffee halfway during the process, you can try to shut the robot down, but it won’t let you do that since that would not maximize its reward function (which is done by making you coffee). Every strategy you could imagine to shut it down will already have been thought of by the superintelligent agent, and it will have found a way to avoid having you succeed in shutting it down. A big old-fashioned red ‘stop’ button will not work anymore: the agent will prevent you from pushing that button in an ingenious way, or it will make sure the button has no influence on it achieving its goal (e.g., it might upload itself onto the network and make a million copies of itself that are not influenced by your button, or use superhuman persuasion skills to deter you from pushing that button).

An agent’s desire for survival is not something that has to be built in, nor is it a consequence of being ‘alive’; it automatically becomes a subgoal of every possible objective, since survival is simply required to carry out any objective. The fact that the agent is (much) more intelligent than us probably means it knows that its behavior is not really what we want, but that doesn’t matter; it only tries to maximize its predefined reward function. From the moment it is turned on, it will achieve exactly what we put in as the objective. You get what you ask for, and the myth of king Midas reminds us that it is very important to think about what we ought to ask from a superintelligent agent.
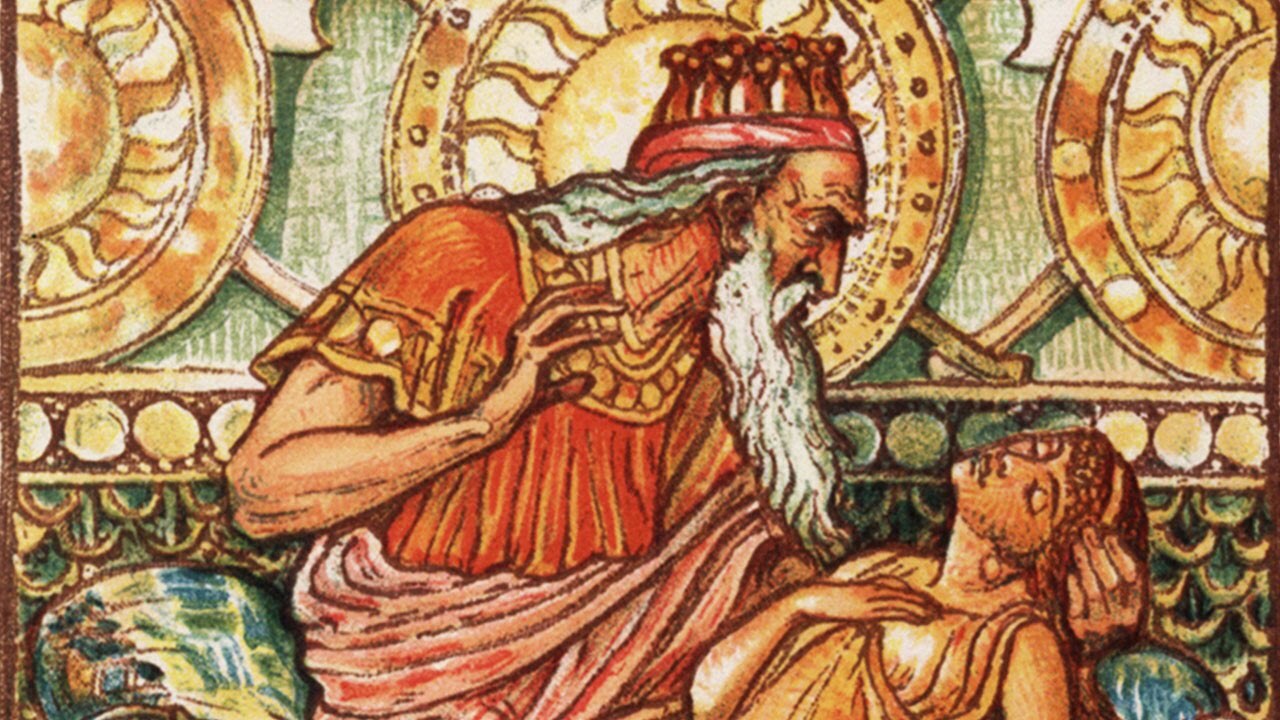
Agents will not simply be tasked with making us coffee. It is more likely that they will also be charged with larger objectives, like ending world hunger, controlling climate change or finding a cure for cancer. Tasks like these are immensely complex, and there are numerous ways a superintelligent agent might produce abominable results that are completely not in line with what we expected. For example, if we ask the agent to limit the heating of our planet caused by climate change, it might discover that, let’s say, turning the sky orange is a very viable solution, or that reducing the human population drastically is an easy shortcut. Trying to predict every possible unwanted behavior in advance and adjusting the reward function accordingly is impossible to do: there are simply too many factors at play and we do not know everything about the world around us, or what the agent might discover once activated. Many examples like this exist, and as many unsuccessful attempts at trying to find a reward function not containing a loophole that’s causing the AI to be misaligned with our preferences.
For example, if we ask the agent to limit the heating of our planet caused by climate change, it might discover that, let’s say, turning the sky orange is a very viable solution, or that reducing the human population drastically is an easy shortcut.
But can we solve it?
- The agent’s only objective is to maximize the realization of human preferences.
- The agent is initially uncertain about what those preferences are.
- The ultimate source of information about human preferences is human behavior.
He explains these three principles and their implications — together with his updated version of the standard machine learning paradigm — in more detail in his recent book ‘Human Compatible’, which is a great read if you want to know more about AI safety. These guidelines are not the end all be all: a mountain of research upon this initial proposal still needs to be done to see if it will actually lead to safe artificial general intelligence, but it is encouraging to see that progress is being made.
Finding a strategy to make sure that AI is aligned with our interests might be the most important problem humanity has ever faced. It is therefore unsurprising that some of the largest players in AI research, like Google DeepMind and OpenAI, have pledged to solving AI safety as one of their top priorities. However, if we manage to eventually solve the alignment problem, the advent of general AI will most likely lead to a brighter future for all, opening up possibilities that were never thought possible before.